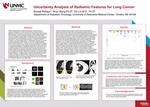
Title
Uncertainty Analysis of Radiomic Features for Lung Cancer
Files
Download Konpal Rafique Original Presentation File (867 KB)
Loading...
Publication Date
Summer 8-6-2020
Faculty Mentor
Dr. Wang and Dr. Lin
Research Mentor
Dr. Wang and Dr. Lin
Document Type
Poster
Abstract
Medical imaging has been an essential component in clinical cancer care. Computer Tomography (CT) is the most used medical imaging modality for diagnosis and treatment response monitoring of a variety of cancer types, including pancreatic cancer. However, the current practice of medical imaging analysis is still mainly intended for visual interpretation and lesion size determination. Recent success in quantitative imaging analysis is redefining the role of medical imaging as a new data source of novel biomarkers, in the form of imaging-based phenotyping. Radiomics is a method of high-throughput extraction of hundreds of features encrypted in the medical images based on a segmentation (delineation of boundary of a three-dimensional volume of interest, i.e. VOI). These Radiomics features typically include the shape, first-, or second- (or textual), and higher-order statistics of a 3D volume of interest, and can provide a far more comprehensive, quantitative, and nuanced representation of the radiographic phenotype of a tumor or an organ, in comparison to the semantic or qualitative descriptors from human experts. Due to its distinct advantages for biomarker development, it becomes an active area of research focusing on risk assessment and treatment response prediction of cancer as well as relationship between image feature and genomics. As previously stated, the inconsistency and variability of target volume delineation and radiomics feature extraction will affect, in a negative way, the robustness of the predictive model and its ability to generate on a different dataset. Patient positioning and image acquisition also affect each feature to varying degrees by introducing different types of imaging perturbations such as image rotation. In this study, we aim at evaluating the uncertainties introduced by the image rotation.
Keywords
Radiomic, Lung Cancer, Medical Imaging
Recommended Citation
Rafique, Konpal; Wang, Shuo; and Lin, Chi, "Uncertainty Analysis of Radiomic Features for Lung Cancer" (2020). Posters: 2020 Summer Undergraduate Research Program. 16.
https://digitalcommons.unmc.edu/surp2020/16
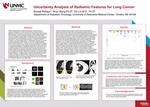